Unleashing the Power of Attention Mechanisms: Fueling Breakthroughs in AI
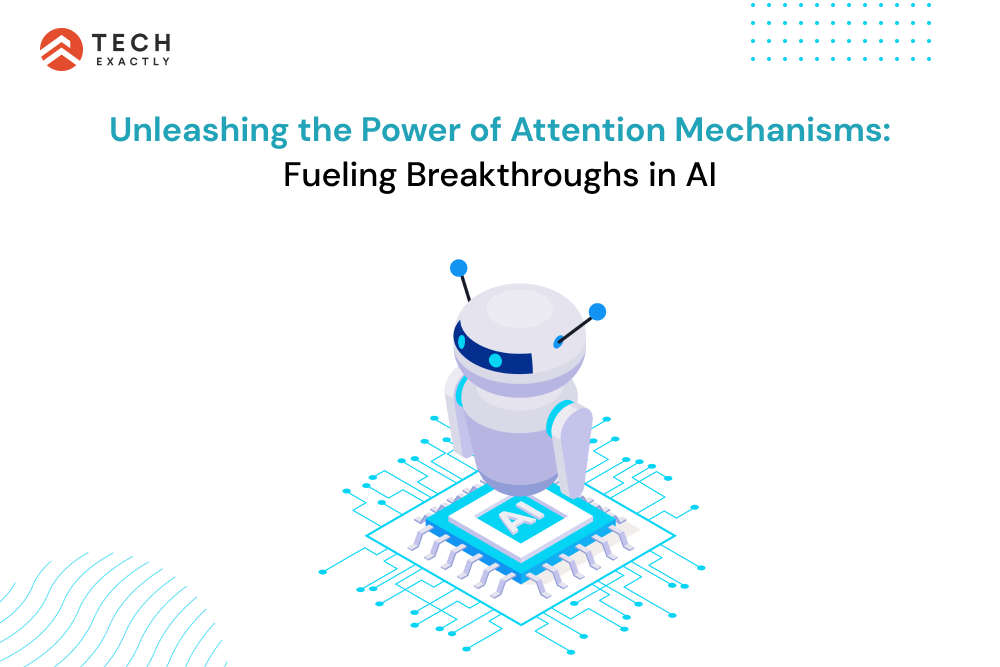
Introduction
Welcome to TechExactly! In today’s article, we are going dig into the interesting world of the Attention mechanism and uncover how it works. Attention mechanisms could be an essential component in present-day profound learning models, empowering them to center on critical data inside a given setting.
One of the key components driving these breakthroughs is the Attention mechanism, a crucial concept in AI that mimics the particular center of human consideration. Below we have provided brief information regarding this topic.
So, snatch a cup of coffee, and let’s plunge into the captivating world of Attention mechanism in deep Learning!
Understanding Attention Mechanisms:
At its center, consideration is the capacity to specifically concentrate on a specific perspective of our environment while overlooking others. This same guideline applies to Attention mechanisms in AI, where models learn to center on pertinent parts of input information (pictures, content, etc.) while ignoring unessential data.
By doing so, Attention mechanisms empower AI models to form better expectations, improve decision-making, and progress in general execution.
Applications in Common Dialect Preparing (NLP):
Attention mechanisms have found far-reaching applications within the field of Common Dialect Handling (NLP), where they have essentially moved forward assignments like machine interpretation, opinion examination, and content summarization.
For occasion, in machine interpretation, Attention mechanisms permit the show to center on the significant parts of the input sentence while creating the comparing interpreted yield. This specific consideration significantly progresses the exactness and familiarity of interpretations, making them more human-like.
Upgrading Computer Vision:
In computer vision, Attention mechanisms have brought approximately exceptional headways in question discovery, picture captioning, and indeed self-driving cars. By specifically going to diverse districts of a picture, AI models can precisely recognize objects, get them set, and create graphic captions.
In self-driving cars, Attention mechanisms offer assistance the AI framework centers on potential deterrents, activity signs, and people on foot, guaranteeing a more secure route.
Unleashing Imagination in Generative Models:
Attention mechanisms have revolutionized the field of generative models, empowering AI frameworks to form practical and assorted yields. In errands like picture era or content era, Attention mechanisms help the model center on important highlights or words, coming about in more nitty gritty and coherent yields.
This has driven breakthroughs in creating reasonable pictures, composing compelling stories, and composing music.
The Longer Term of Attention Mechanisms:
As AI proceeds to evolve, attention components are anticipated to play an indeed more basic part in future breakthroughs. Analysts are exploring ways to improve Attention mechanisms, making them more strong and interpretable.
Modern attention-based models are being created to handle complex assignments like multi-modal learning, where AI frameworks can prepare and get data from different sources at the same time.
In any case, Attention mechanisms are not without their challenges. Preparing attention-based models can be computationally costly, requiring noteworthy handling control and information.
Also, the interpretability of Attention mechanisms remains a continuous inquiry about the region, as understanding how AI models arrive at their decisions is crucial for building belief and straightforwardness.
Attention mechanisms are an energizing concept in AI that closely mirrors the specific center of human consideration. By empowering AI models to concentrate on significant data, Attention mechanisms have fueled breakthroughs in different spaces, from NLP to computer vision.
As Attention mechanisms proceed to development, we will anticipate more momentous developments in AI, upgrading our lives and driving us toward a future where AI and human insights consistently coexist. So, keep your eyes peeled (quip planning) for the next wave of attention-fueled breakthroughs in AI!
How Attention Mechanism Works
The concept of consideration starts from human cognitive forms. When we see the world, our brain specifically goes to particular jolts while overlooking others. Additionally, in fake insights and common dialect preparing (NLP) assignments, the Attention mechanism permits models to specifically center on pertinent parts of the input grouping.
It makes a difference that models keep in mind and utilize past data successfully, driving to made strides in execution in assignments such as machine interpretation, picture captioning, and content summarization.
The Key Players: Encoder, Decoder, and Consideration
At the heart of Attention mechanisms lies the trio of encoder, decoder, and consideration. Permit me to break them down for you:
-
Encoder:
The encoder is mindful of changing the input information into a more unique representation. For occurrence, in dialect interpretation, the encoder changes over a sentence into a grouping of numerical vectors, capturing the semantic meaning.
-
Decoder:
The decoder, as the title recommends, interprets the encoded representations produced by the encoder. In interpretation errands, the decoder takes the encoded vectors and creates the deciphered sentence. Straightforward, right?
-
Consideration:
Here comes the star of the appear! Consideration, in its least difficult shape, calculates the pertinence or significance of diverse parts of the input for a given assignment. It makes a difference that the decoder centers on the foremost important data at each step, coming about in more precise interpretations or classifications.
Attention Instrument in Profound Learning
Profound learning models, such as repetitive neural systems (RNNs) and transformers, use the Attention mechanism in deep Learning to improve their execution. To understand how consideration works, let’s consider the case of a machine interpretation errand, where a show must translate a sentence from one dialect to another.
-
Encoding the Input:
The source sentence is first encoded into a numerical representation utilizing strategies like word embeddings or character embeddings. This representation permits the model to understand the meaning and setting of the input.
-
Consideration Scores:
Consideration scores are calculated by comparing each word or token within the source sentence to each other word or token. These scores show the significance or relevance of each word within the setting.
-
Softmax Normalization:
The consideration scores are at that point normalized employing a softmax work. This step guarantees that the scores sum up to 1, making them interpretable as a likelihood dispersion.
-
Weighted Setting:
Each word within the source sentence is increased by its corresponding consideration score, giving more weight to critical words. The weighted words are at that point combined to make a setting vector that represents the important data within the source sentence.
-
Translating the Yield:
The setting vector, besides the already created yield, is bolstered into the decoder of the model, which creates the interpreted sentence word by word. The Attention mechanism makes a difference the demonstration centers on the important parts of the source sentence while generating the yield.
Benefits of Attention Mechanism
The Attention mechanism offers a few benefits that contribute to the victory of profound learning models:
-
Improved Demonstrate Execution
The models with Attention mechanisms are regularly way better able to capture the connections between diverse parts of the input arrangement. This leads to forward execution in different NLP assignments, counting machine interpretation, opinion examination, and discourse acknowledgment.
-
Interpretability
Consideration scores give bits of knowledge into which parts of the input are considered more imperative by the show. This interpretability permits analysts and professionals to get the thinking behind a model’s expectations and alter them as required.
-
Efficient Computation
Attention mechanisms are computationally effective compared to conventional strategies that consider all parts of the input similarly. By specifically going to significant parts, models diminish repetition and center computational assets on the foremost instructive fragments.
-
Later Progresses and Developments
Over the long time, Attention mechanisms have advanced and been refined to address particular challenges. One eminent advancement is the presentation of self-attention components in transformer models. Self-attention permits the model to go to diverse positions within the input arrangement to capture conditions and long-range connections more successfully.
Moreover, analysts are exploring attention instruments past NLP errands. Attention-based models have appeared guaranteed in different spaces, such as computer vision, where they help in question location, picture classification, and picture captioning errands.
Attention mechanisms have revolutionized the field of profound learning, empowering models to center on pertinent data and move forward with their execution in complex assignments. By specifically going to critical parts of the input, Attention mechanisms improve the model’s capacity to get it set and create precise expectations.
As the field progresses, Attention mechanisms are likely to play a progressively significant part in different spaces of counterfeit insights.
Why Select TechExactly for Attention Mechanism in Profound Learning
Are you prepared to jump into the captivating world of profound learning? On the off chance that you’re trying to find an effective instrument to upgrade your deep learning models, at that point, you’ve come to the proper put.
Nowadays, we’re progressing to conversation almost why you ought to select us for the Attention mechanism in deep Learning.
So, let’s buckle up and get begun!
To begin with, things, first, let’s rapidly revive our memory of what the Attention mechanism is. Within the domain of profound learning, the Attention mechanism permits the demonstrate to focus on pertinent parts of the input arrangement, giving more weight to critical data and less weight to unimportant points of interest.
It’s like a highlight that lights up the foremost pivotal perspectives of your information. Lovely slick, huh? Presently, let’s turn our consideration (play on words expecting!) to why we are the idealize choice for the Attention mechanism in profound learning.
-
Expertise:
Here, we have been at the cutting edge of innovation for a long time. Our expert team members use a wide range of knowledge and make sure that it will produce a better result. With us, you’ll be able to rest assured that your models are in able hands.
-
State-of-the-Art Strategies:
Here we are continually pushing the boundaries of profound learning investigations. Our group remains up-to-date with the most recent progressions within the field and joins state-of-the-art procedures into our Attention mechanism arrangements.
By choosing us, you’ll have got to cutting-edge innovations that provide your models a competitive edge.
-
Customization and Adaptability:
Here, we get that each extension is one of a kind. That’s why we offer customizable Attention mechanism arrangements custom-fitted to your particular needs. Whether you’re working on picture classification, normal dialect preparation, or any other profound learning assignment, ready to adjust our attention mechanism to fit your prerequisites like a glove.
-
Consistent Integration:
We do not need you to hop through bands to incorporate our Attention mechanism into your existing workflows. Our arrangements are outlined to be consistently coordinated with well-known profound learning systems like TensorFlow and PyTorch.
With us, you’ll be able to effortlessly upgrade your models without causing superfluous migraines.
-
Proficient and Versatile:
Time is of the quintessence within the tech world. We get it that you just require effective and versatile arrangements to meet your due dates. Our Attention mechanism is optimized for execution, permitting you to prepare huge sums of information rapidly and successfully.
Whether you’re working to a small extent or taking care of gigantic datasets, we have got you secured.
-
Bolster and Collaboration:
We accept building solid connections with our clients. After you select us, you’re not fair getting an item; you’re getting an accomplice. Our devoted support team is continuously prepared to help you and address any concerns or questions you will have.
We’re here to assist you to succeed in your profound learning endeavors. So, there you have got it! Six compelling reasons why we are the go-to choice for attention component in profound learning.
With our ability, state-of-the-art methods, customization options, seamless integration, proficiency, and support, we’ve got all the fixings to take your profound learning models to new heights. Keep in mind, that choosing the proper tools and innovations can make all the difference within the world of deep learning.
Conclusion
With us by your side, you will be able to tackle the control of the Attention mechanism and open the complete potential of your models.
Do not miss out on this extraordinary opportunity! Prepared to take your profound learning ventures to the next level? Head over to TechExactly now and find the enchantment of the Attention mechanism in deep Learning. We can’t wait to connect with you on this energizing travel!